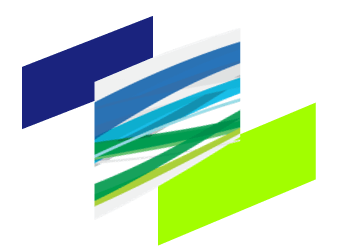
BLOG
Variant Filtering vs. Algorithimic Prioritization for Genome Interpretation
Genomics provides the opportunity to realize the promise of personalized medicine, with tests scaling from single genes to comprehensive panels, exomes and whole genomes. Whole genome and whole exome sequencing provide a wealth of information to potentially identify the cause of disease in an individual. In fact, a single exome has tens of thousands of variants.
Traditional variant interpretation methods of prioritization and filtering are often underpowered to effectively analyze these large sets of variants, however, and when analyzing individuals and nuclear families many cases still go undiagnosed.
Variant Prioritization and Filtering
Filtering techniques apply hard cut-offs to data. Often applied progressively or in parallel workflows, filters can be time-consuming and difficult to track. Filtering can result in premature or inadvertent removal of causative candidates from the consideration set, and at the same time may also generate large numbers of false-positive candidates.
Prioritization algorithms typically evaluate variants based on predicted deleteriousness. Prioritization is driven by individual variants and may be limited to coding regions resulting in incomplete coverage. Traditional bioinformatic variant prioritization algorithms often only look at amino acid impact or conservation at a specific position, and do not look at allele frequency or aggregate impact at a gene level.
A More Comprehensive Approach to Variant Analysis
A more comprehensive approach is required to accurately interpret whole exome and genome data from individuals and nuclear families. VAAST ranks variants and their associated genes by their likelihood to cause disease in a specific individual, family, or cohort. VAAST evaluates predicted impact on protein function, allele frequency as well as evolutionary conservation in its statistical ranking process. This is superior to traditional techniques that look at individual variants only or that only look at one or a subset of these factors using filtering approaches.
VAAST was first published in 2011 when it was used to discover the genetic cause for Ogden Syndrome [2]. This was one of the first disease gene discoveries using next-generation sequencing (NGS). VAAST has since been used in numerous cases to identify the underlying genetic cause of disease, for both known and novel disease genes.
Algorithmically Integrating a Patient’s Phenotype Data to Refinance Prioritization
Phevor™ (Phenotype Driven Variant Ontological Re-ranking tool) integrates a patient’s or a cohort’s phenotypic information into a comprehensive clinical bioinformatics genome analysis.
Phevor, published in 2014, uses a novel algorithmic approach to directly integrate clinical phenotype information with gene function and disease information – bridging the gap between clinicians and computational biologists3. Phevor starts by mapping phenotype terms to the Human Phenotype Ontology4, Gene Ontology and other ontologies then uses a unique network propagation approach to identify additional gene candidates. This process creates a ranked list of genes ordered by the specific phenotype provided. Phevor then combines this prioritized list of genes with the VAAST analysis to produce a combined ranking of candidate genes based on deleteriousness and the specific phenotype or phenotypes in question.
The 2014 Phevor paper outlines three cases where Phevor was used to ascertain the genetic cause of disease in three undiagnosed children, including identification of a novel disease gene in a 6-month old infant with idiopathic liver disease. The integration of VAAST and Phevor within the Fabric Enterprise platform provides intuitive access to these advanced algorithms, and usage within Fabric Enterprise’s clinical interpretation workflows. Fabric Enterprise also allows users to combine these advanced algorithms with traditional filtering techniques, accelerating and improving the accuracy of interpretation while providing flexibility.
This combined algorithmic variant interpretation approach significantly increases the power and likelihood for diagnosis in individual patients or patients with two or three other family members, the most commonly occurring clinical scenarios.
Fabric’s latest gene-ranking algorithm, GEM, builds on VAAST and PHEVOR and incorporates clinical information from OMIM and ClinVar. GEM uses novel Artificial Intelligence (AI) methods to dramatically improve the speed, efficiency, and effectiveness of clinical genome interpretation. GEM takes as inputs the clinical features of the patient along with their genome sequence and produces a concise ranked list of clinically relevant variants. Benchmarking data across several cohorts of previously diagnosed rare genetic disease patients has shown the algorithm to be highly sensitive for finding known disease genes, with the causal gene ranking in the top 2 over 90% of the time. GEM consistently outranks other algorithms in its ability to detect causal variants while reviewing the smallest number of genes.  GEM is positioned to dramatically increase the speed and efficiency of clinical genome analysis, with applications in the analysis of undiagnosed rare disease, rapid analysis of NICU/PICU cases and routine re-analysis of negative genomes.
References
[1] Adzhubei et al, Nat Methods 2010.
[2] Rope et al, Am J Hum Genet. 2011, Yandell et al, Genome Res. 2011
[3] Singleton et al, Am. J. Hum. Gen. 2014
SUBSCRIBE